Prof. Heiko Balzter, Director of the Institute for Environmental Futures, University of Leicester
1. The role of agriculture, forestry and other land use in global climate action
Agriculture, Forestry and Other Land Use (AFOLU in IPCC jargon) accounted for 13–21% of global total anthropogenic greenhouse gas (GHG) emissions from 2010 to 2019 according to the IPCC Sixth Assessment Report (medium confidence). After accounting for managed and natural terrestrial ecosystems as carbon sinks, an estimated net CO2 emission of +5.9±4.1 GtCO2yr–1 remains.
The COP28 UAE Declaration on Sustainable Agriculture, Resilient Food Systems, and Climate Action resolved to ‘maximize the climate and environmental benefits – while containing and reducing harmful impacts – associated with agriculture and food systems by conserving, protecting and restoring land and natural ecosystems, enhancing soil health, and biodiversity, and shifting from higher greenhouse gas-emitting practices to more sustainable production and consumption approaches, including by reducing food loss and waste and promoting sustainable aquatic blue foods’. It included a commitment of world leader to integrate agriculture and food systems into national climate action plans and to mainstream climate action across policies and actions related to agriculture and food systems.
In the UK, about 11% of net GHG emissions originate from agriculture and other land use. The main sources include beef and dairy farming as sources of methane and other GHGs (around 7%) and carbon emissions from drained peatland (around 4%, Figure 1). While emissions from forestry and other land use have seen a substantial reduction of over 90% since 1990 in the UK, agricultural emissions remain high and have only declined by 12% since then.
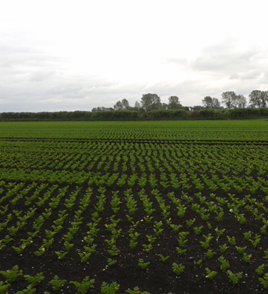
Figure 1: A cultivated drained peatland field in The Fens, East Anglia. This production system was a net CO2 source of 760 gC m-2yr-1 as measured by an eddy covariance flux tower.
One of the main challenges in enabling the transition of the agriculture sector towards net zero is that many farmers are understandably reluctant or unwilling to give up production in exchange for the financial government incentives. It is also not politically desirable to give up food production on a large scale and risk further food price inflation.
The tension between the desire and the need for maintaining food production to increase food security on one side, and reducing GHG emissions from the production systems on the other side, makes this problem difficult to resolve. Digital twins and AI offer a likely solution to this fiendishly complex problem.
How Environmental Digital Twins can accelerate the transition to net zero
A digital twin is a self-updating digital representation of a physical environmental system. It continuously ingests new data streams and model simulations to reflect the current state of the physical twin and is able to create scenario simulations to aid decision-making. The human decision-maker is empowered to make better-informed decisions based on enhanced AI-enabled insights into the environmental system behaviour. With these three components, the creation of the digital twin and embedding it in an operational decision-making context has the power to transform the physical world, or in our case the environment.
Funded by EPSRC and UKRI, our team of researchers from the Universities of Leicester, Loughborough and Bristol are developing self-learning digital twins for ruminant farming (beef and dairy) and cultivated drained peatlands, the two main emission sources from AFOLU in the UK. Our digital twins use AI-powered models of GHG fluxes. Users of the digital twin can explore impacts of their land use or policy decisions and how resilient they are to future climate change impacts. This way, we intend to provide actionable insights for achieving the UK’s legally binding net-zero targets of 81% emissions reduction by 2035, as announced by Prime Minister Sir Keir Starmer at COP29 in Baku, and net zero by 2050.
The paradigm of self-learning digital twins
What makes a digital twin self-learning? Traditional digital twins are often based on trained AI models that do not adapt to new situations without a human retraining the models. The self-learning functionality enables the digital twin to confront its own model simulations with new observational data on a regular basis and without human involvement. It is then able to assess whether the model still behaves as expected or whether it is beginning to drift away from the new observations. The digital twin is then formulating modifications to its AI models that improve the simulations. An optional step can be that the modifications can be checked and agreed by a human supervisor.
Prototype digital twins for sustainable land management
The Peatlands Digital Twin developed by the University of Leicester is enabled by real-time AI and integrates JULES-Crop land surface model simulations, Earth observation data from satellites, eddy covariance flux tower data from field stations, climate data, and land use information at field-scale to deliver actionable insights to farmers and policy-makers (Figure 2). It is learning continuously from incoming data, enabling ongoing predictions of emissions and land use outcomes. The Digital Twin aims to support farmers and policy-makers in making decisions that reduce GHG emissions and promote sustainable and resilient agriculture. The self-learning digital twin is powered by a directed graph neural network and a random forest model.
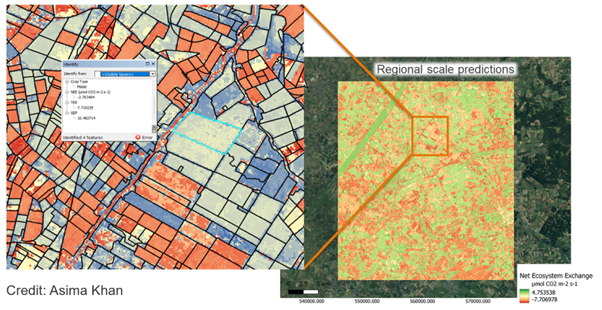
Figure 2: Carbon dioxide flux map at field scale showing Net Ecosystem Exchange (NEE) simulations of cultivated drained peatland in The Fens, East Anglia from an Earth observation model trained on eddy covariance flux tower measurements at field scale. Such maps can be produced automatically every week or two based on new data.
The Ruminant Digital Twin developed by Loughborough University has an AI-powered model for reducing GHG emissions from ruminant farming at its core. It has been trained on farm-scale and animal-scale measurements. The digital twin highlights GHG emissions, particularly methane, and includes satellite observations at national scale in relation to land use and seasonal variations. Its machine learning and state-of-the-art deep learning is intended to assist farmers in predicting milk yield, ammonia emissions (which is a significant air pollutant), methane and nitrous oxide emissions, influenced by decisions on animal feed, pasture management practices, land use as well as environmental and climate factors. Users have the flexibility to adjust a range of parameters, select various factors, and receive predictive insights.
The digital twins are intended to support cost-effective, informed decision-making and optimise practical strategies to advance toward net zero emissions. They can inform sustainable decision-making on farm-scale as well as the development of environmental land use policies in the UK.
Users interact with the digital twins via a graphical user interface (GUI) and can explore how their management choices (crop types, sowing dates, water table depth, herd management etc.) and future climate impacts are likely to influence their GHG emissions and crop/milk yields.
Watch the Gemini Call Presentation
Want to see how these insights were originally shared with the Digital Twin Hub community? Watch my Gemini Call presentation where Heiko delivered his presentation:
Call for Speakers: Share your Digital Twin story in 2025
We’re actively seeking presenters who can contribute to our community’s knowledge and understanding of Digital Twins. Whether you’re working on groundbreaking projects, conducting research, or have valuable lessons learned to share, we want to hear from you.
Ready to share your expertise? Complete our quick 10-minute speaker proposal form:
Join the community in advancing the conversation around Digital Twins and their transformative potential across industries.
Further material
Gemini Call Live: https://www.youtube.com/watch?v=o1T5CaygNZU
AI4NetZero Project: https://le.ac.uk/research/institutes/environmental-futures/projects/ai4netzero
AI4Net Zero Webinar: https://www.youtube.com/watch?v=AOxZCVuQi80
Acknowledgement of our co-investigators and project partners:
- Loughborough University
- University of Bristol
- Bloc Digital
- CGI
- FNK Designs
- Geospatial Insight
- MAXAR
- Planet Labs
- National Centre for Earth Observation
- Hooks Farm Dairy
- National Bovine Data Centre
- Cattle Information Service
- Silvasheep
Leave a comment