Download File
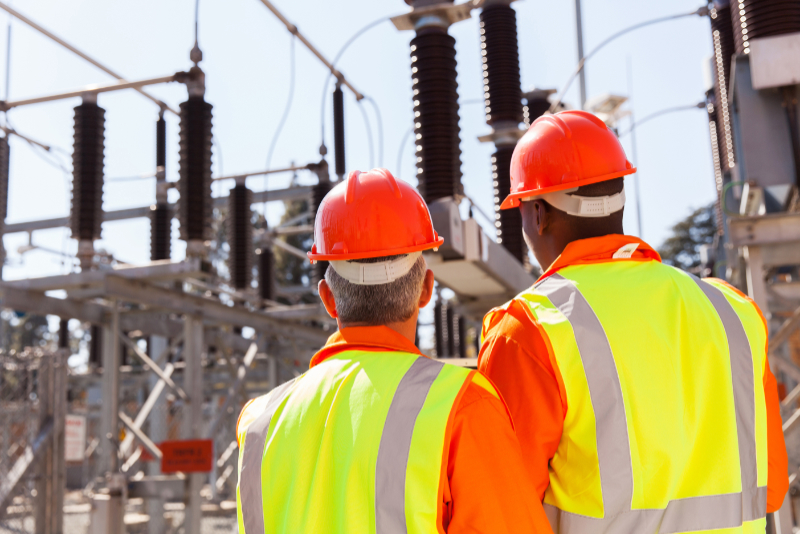
- File Information
- Size: 1.7MB
- Type: pdf
Summary
Planning for resilience against climate hazards in our economic infrastructure requires a systems approach. The development of connected digital twins that enable the sharing of information between infrastructure sectors and organisations will improve our understanding of how assets fail, where they fail and how any cascading failures can better inform resilience planning at the systems-level. To understand the connections between different assets in a system — and how a cascading failure could impact service provision — the vulnerability and probability of failure of individual assets and failure modes against different climatic hazards need to be understood.
In this use case, the vulnerability of individual assets within an infrastructure system has been considered as the susceptibility of an asset to failure, its condition, capacity and ability to cope in the presence of the hazard, and it is expressed in probabilistic terms. Thus, asset failure following exposure to coastal flooding has been assessed using probabilistic modelling. This allows for the consistent interrogation of how individual assets in the system could fail under various incidents induced by coastal flooding. Singular characteristics of some assets and asset networks require distinct probability distributions that consider specific causal pathways that would lead the asset to fail. Bayesian Network Modelling uses probabilities to represent all uncertainties that are quantified for the modelling, and was adopted as the common language to model probability of failure and express vulnerability caused by coastal flooding at the asset-level.
This report outlines the general approach undertaken in the connected digital twin to assess the probability of failure of assets within the network and how it has been applied specifically to investigate the probability of failure of an individual wastewater pumping station against coastal flooding.
The model for the probability of failure requires input in the form of flood information (extent, depth), supported by expert judgement. This is to understand the causal pathways leading to the failure of an asset in the example of a wastewater pumping station. The development of a probabilistic model that is sufficiently realistic to deliver the intended goal is paramount for understanding vulnerability at the asset level and how this can affect the wider infrastructure system. This requires elicitation from experts in asset owner/manager organisations. Engaging with experts who operate individual assets not only provides a detailed understanding of the functioning /non-functioning of an asset, it also allows investigation of probabilities of failure for individual asset components under various operating conditions and past incidents in collaboration with asset owners. Through this engagement, a calibration of the probabilistic models can be undertaken for various sites and asset classes.
The work conducted focusses on how Bayesian Network Modelling can be implemented across the asset network, by understanding and integrating a range of failure modes into a working interface. Key to the failure of an asset is the direct exposure of its main parts to coastal flooding. Elements such as the submergibility of parts, their location above the standard level of protection of the asset or how the asset operates during increasing flows in the wastewater network as a result of the coastal flooding conditions would have an impact on any direct failure assessment. Other indirect failure modes highlighted in the findings originate from externalities to individual assets, including its accessibility and thus its reliance on transport infrastructures to implement pre-incident and post-incident recovery efforts. Similarly, the dependence of an asset on external power supply and telecommunication provision for its functioning could lead to its failure if such external connections were cut prior or during a flood incident.
The modelling work conducted in this phase of work considers maximum flooding conditions occurring at the same time across the entire network and leading to the worst disruption. It is recommended that future asset vulnerability modelling should focus on both the asset-specific and external factors that influence the probability of failure, allowing for the sequencing of failures as the flood event unfolds and alongside other associated climatic hazards. Investigations of how quickly an asset can recover after failing is another aspect that should be taken into account in the evaluation of its vulnerability. This would further support the system-wide vulnerability and cascading failure assessments.
Overall, the deployment of Bayesian Network Modelling across the network demonstrates the benefits of having an overarching framework for assessing vulnerability of individual assets. Such assessments could be improved by investigating different combinations of failure modes across assets, and testing probability of failures across multiple climate scenarios, within the same interface. Whilst such an approach allows for a level of fine-tuning to calibrate site-specific probability distributions, the greatest benefits to resilience and investment planning decisions would be to implement the approach at the systems level. This would involve a multi-sectoral network of hundreds to thousands of assets.
Â
Â
Â